A lot of PC gamers were wondering whether AMD’s solution to NVIDIA’s DLSS tech would be using similar AI techniques. And today, we finally have some details about AMD’s Super Resolution. According to a patent that was filled by the red team, AMD Super Resolution will be using AI neural networks in order to improve the image.
In its patent, AMD explains that the conventional super-resolution techniques are not that great. According to the red team, these solutions result in blurry and/or corrupted images. Thus, AMD promises to provide devices and methods for efficiently super-resolving an image, which preserves the original information of the image while upscaling the image and improving fidelity. The devices and methods utilize linear and non-linear up-sampling in a wholly learned environment.
The AI neural network which AMD calls “GSR” will efficiently super resolve images in a convolutional and generalizable manner. The GSR architecture will render games at lower resolutions and upscale them to higher resolutions. Similar to DLSS, it aims to create high-quality image approximations and achieve high framerates.
Here are the most interesting parts from AMD Super Resolution’s patent.
Gaming Super Resolution
A processing device is provided which includes memory and a processor. The processor is configured to receive an input image having a first resolution, generate linear down-sampled versions of the input image by down-sampling the input image via a linear upscaling network and generate non-linear down-sampled versions of the input image by down-sampling the input image via a non-linear upscaling network. The processor is also configured to convert the down-sampled versions of the input image into pixels of an output image having a second resolution higher than the first resolution and provide the output image for display
[0008] Conventional super-resolution techniques include a variety of conventional neural network architectures which perform super-resolution by upscaling images using linear functions. These linear functions do not, however, utilize the advantages of other types of information (e.g., non-linear information), which typically results in blurry and/or corrupted images. In addition, conventional neural network architectures are generalizable and trained to operate without significant knowledge of an immediate problem. Other conventional super-resolution techniques use deep learning approaches. The deep learning techniques do not, however, incorporate important aspects of the original image, resulting in lost color and lost detail information.
[0009] The present application provides devices and methods for efficiently super-resolving an image, which preserves the original information of the image while upscaling the image and improving fidelity. The devices and methods utilize linear and non-linear up-sampling in a wholly learned environment.
[0010] The devices and methods include a gaming super resolution (GSR) network architecture which efficiently super resolves images in a convolutional and generalizable manner. The GSR architecture employs image condensation and a combination of linear and nonlinear operations to accelerate the process to gaming viable levels. GSR renders images at a low quality scale to create high quality image approximations and achieve high framerates. High quality reference images are approximated by applying a specific configuration of convolutional layers and activation functions to a low quality reference image. The GSR network approximates more generalized problems more accurately and efficiently than conventional super resolution techniques by training the weights of the convolutional layers with a corpus of images.
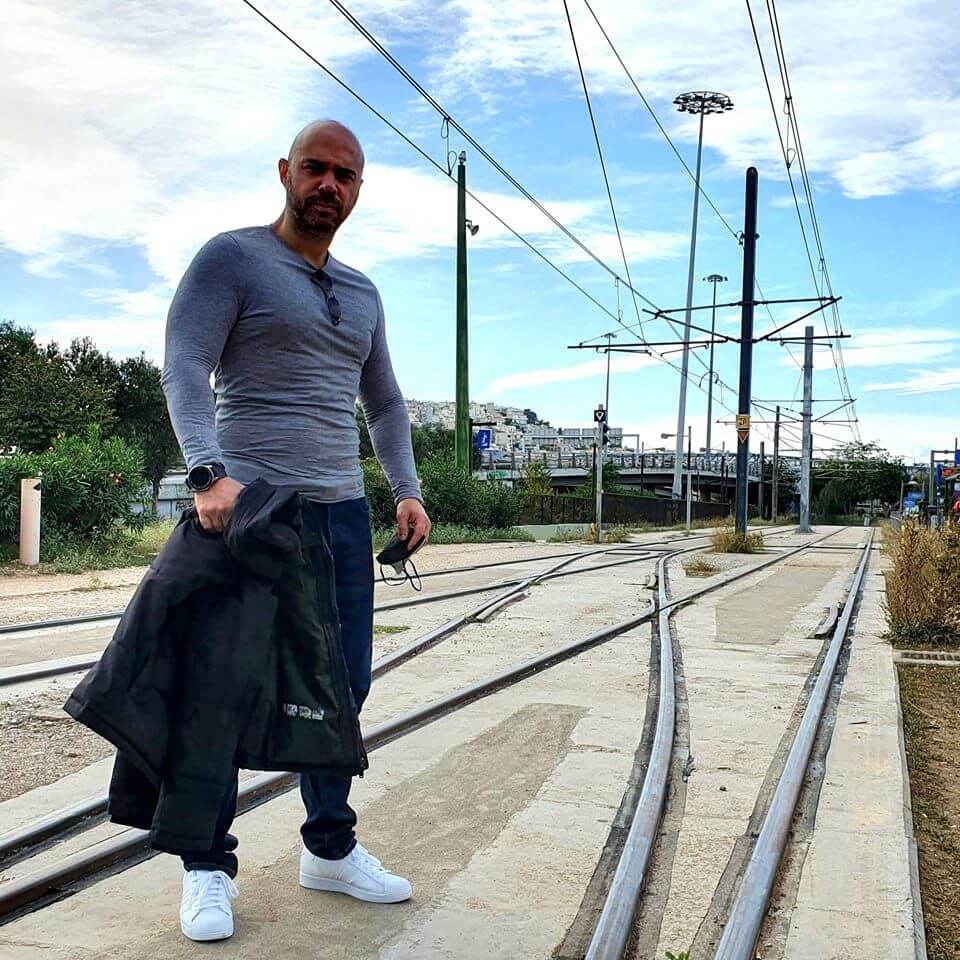
John is the founder and Editor in Chief at DSOGaming. He is a PC gaming fan and highly supports the modding and indie communities. Before creating DSOGaming, John worked on numerous gaming websites. While he is a die-hard PC gamer, his gaming roots can be found on consoles. John loved – and still does – the 16-bit consoles, and considers SNES to be one of the best consoles. Still, the PC platform won him over consoles. That was mainly due to 3DFX and its iconic dedicated 3D accelerator graphics card, Voodoo 2. John has also written a higher degree thesis on the “The Evolution of PC graphics cards.”
Contact: Email